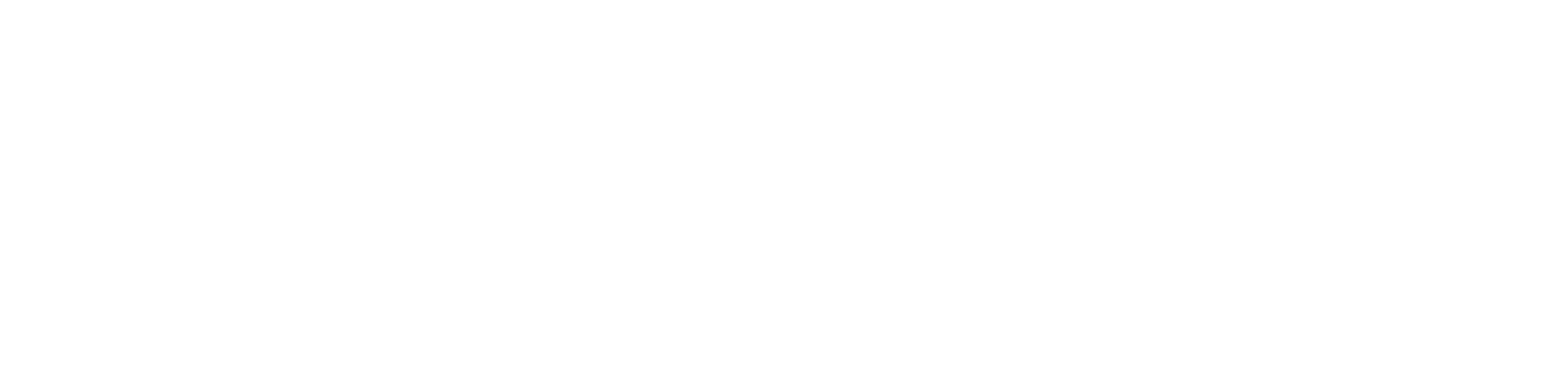
Designed experiments for more understanding and maximum impact.
- Understand cause and effect using the power of statistically designed experiments -- even when you have limited resources.
- Design efficient experiments to meet your real-world constraints, process limitations and budget with the Custom Designer.
- Use a Definitive Screening Design to help you untangle important effects when you have many factors to consider.
Textbook Designs (Classical Designs)
- Full factorial
- Fractional factorial
- Response surface (RSM)
- Blocking designs (RCBD, CBD, IBD, RIBD)
- Split-plot design
- Strip-plot design
- Mixture design
Optimal Designs
- Custom design
- Optimality criteria
- Constrained designs
Screening Designs
- Definitive screening
- Fractional factorial
Robust Designs
- Taguchi array
- Taguchi design
- Noise factor
DOE Concepts (DOE Techniques)
- Randomization
- Replication
- Center points
- Blocking
- Sequential experimentation
- Augment design
Special Purpose Designs
- Space filling
- Covering array PRO
- Choice
- MaxDiff
- Nonlinear design
Evaluate Design
- Design comparison
- Design explorer
- Design diagnostics
- Power
- Aliasing
From the beginning, we were able to quantify how much value DOE adds for us. We were using DOE in several internal cases and we were able to actually quantify our savings of at least 50% of the time and resources. In some cases it was even 70%.
Pilar Gomez Jimenez
Principal Scientist, Johnson Matthey
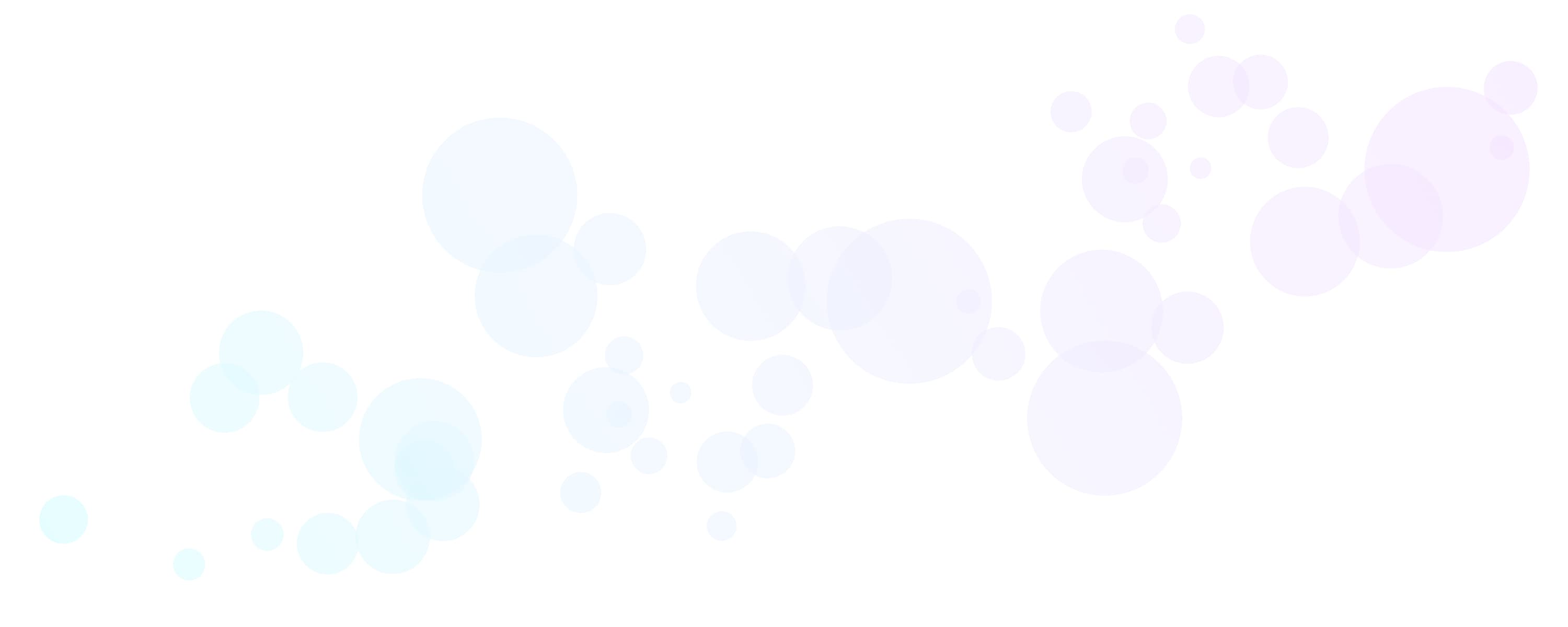
JMP® Analytic Capabilities
See everything that JMP® can do for you and your organization, from data access and cleaning, to exploration and visualization, all the way through sharing and communicating your results.
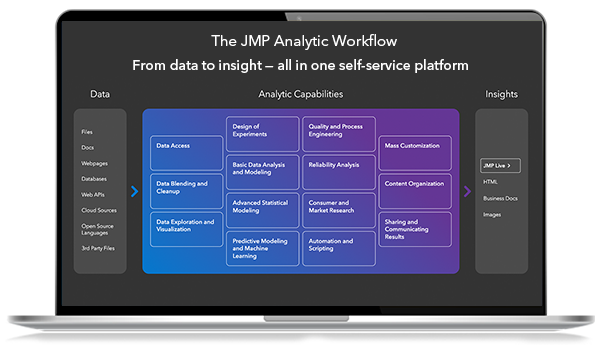