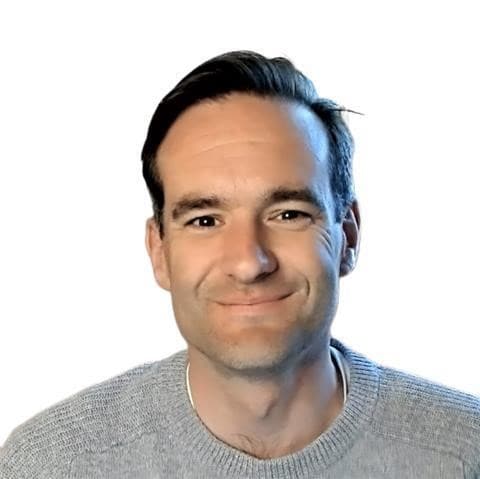
Phil Kay
Phil Kay is a learning manager for JMP Statistical Discovery, a subsidiary of SAS. His job is to understand the science and engineering challenges and provide guidance on data analytic solutions for industrial organisations around the world.
Previously, Phil was a key scientist in the development of numerous processes for the manufacture of colorants for digital printing at FujiFilm Imaging Colorants. Phil has a master’s degree in applied statistics with a dissertation on Design of Experiments. He also has a master’s and PhD in chemistry.
He is a Fellow of the Royal Statistical Society, a Chartered Chemist, and a member of the committee for the Process Chemistry and Technology Group with the Royal Society of Chemistry.
Phil loves showing people how data analytics enables better science. Follow Phil Kay, Evangelist for Data Analytics, on LinkedIn.
When I started as a development chemist in industry, a good day for me was making some stuff that was better than the stuff I had made the day before. That was my idea of success. With time, however, I realised that the product of my experiments wasn’t really the stuff in the flasks, it was the stuff I was learning: the knowledge needed to consistently manufacture products that meet the demands of our customers. It was an epiphany. My job was not about making things, it was to generate understanding.
I expect that there are plenty of chemists right now that have barely begun this journey. To be successful in the digital future of chemistry, scientists will need guidance and encouragement to make that change. We might even be able to avoid the making-stuff mindset taking hold in the first place.
From preps to plans
At school and university, my only experience of experiments was lab practicals. These were useful in demonstrating the synthetic routes that we were learning about and for building practical skills, but success here was often defined as a heap of large, colourless crystals. That left me thinking that experiments were about confirming our understanding of chemical mechanisms.
I was following the scientific method … but there was little in the way of strategy
This notion was challenged immediately in my first job, as a process chemist making toner for laser printing. The system was just too complex and messy to be understood from theory alone - curly arrows were no use! Instead I spent my days painstakingly carrying out ‘preps’ to figure out how to combine latex, pigment dispersion and other ingredients to produce uniformly sized toner particles. With each prep, I would tweak the recipe. I might swap out a raw material, modify concentrations and proportions, or change the temperature of one of the hold phases. There was always a rationale, and I was happy that I was following the scientific method (form a hypothesis, make a prediction, test the prediction), but there was little in the way of strategy. When I arrived at something that worked, I didn’t really know how I had got there. I became accustomed to the unpredictability of this approach, but I never got comfortable with it.
Mind over matter
Then I heard a seminar by statistics professor Dick de Veaux that introduced me to a new way of thinking: the data gathered from observations accumulated over many runs of an industrial process can be ‘mined’ for insights about the behaviours driving the system and to build a model of how the inputs will affect the outcomes. In short, rather than taking one step at a time hoping to end up at the best recipe, I could use the data holistically to make a map that shows me where to find the best recipe.
At least that was the theory. In practice, my own datasets were not very useful. Each prep took significant time and resources, so twenty rows of data was a lot for one project, and that is nobody’s idea of big data. There was little or no common ground between one project and the next, so it was not worth the effort of pulling together historical data. And when I looked at the data, many of the input variables had barely been varied at all. I needed a new mindset to go with the new approach.
It’s never too early nor too late to learn these skills
I needed to plan each project to deliver the data that would help me meet the development objectives. I needed to choose the inputs to vary and the outputs to measure, and how to systematically vary the inputs to optimise what I could learn from each run. Thankfully there was already a methodology for this: Statistical Design and Analysis of Experiments or DOE.
With these tools and my new outlook, my projects became more efficient and predictable, and my colleagues started to pay attention. We had some great successes, such as doubling the productivity of a bottleneck manufacturing step without needing expensive new plant infrastructure. Helping my colleagues with DOE became my full-time passion and professional focus.
I have since learned that many scientists and engineers have gone through similar journeys of realisation. In a recent webinar with Chemistry World, Pilar Gómez Jiménez, a principal scientist at Johnson Matthey discussed how introducing this smarter approach to experimentation helped deliver a 50% reduction in R&D costs for the business. ‘Every chemical problem or every challenge that my colleagues present to me, I see it as a table with columns and rows. That is a total change in mindset…’ she explained.
Like many others, I wish I had learned these ideas earlier in my career. The graduate training in DOE at the Centre for Rapid Online Analysis of Reactions (Roar) at Imperial College is a rare example of bringing these ideas to students before they join industry. But why not have simple DOE exercises for undergraduates or even in high school science classes?
It’s never too early nor too late to learn these skills, and you can start right now. Sign up for our online workshop, Your guide to solving complex problems by mastering Design of Experiments and begin the journey. Stop making stuff and you’ll start making sense.
Let's stay connected!
You may contact me by email regarding news, events and offers from JMP. I understand I can withdraw my consent at any time.