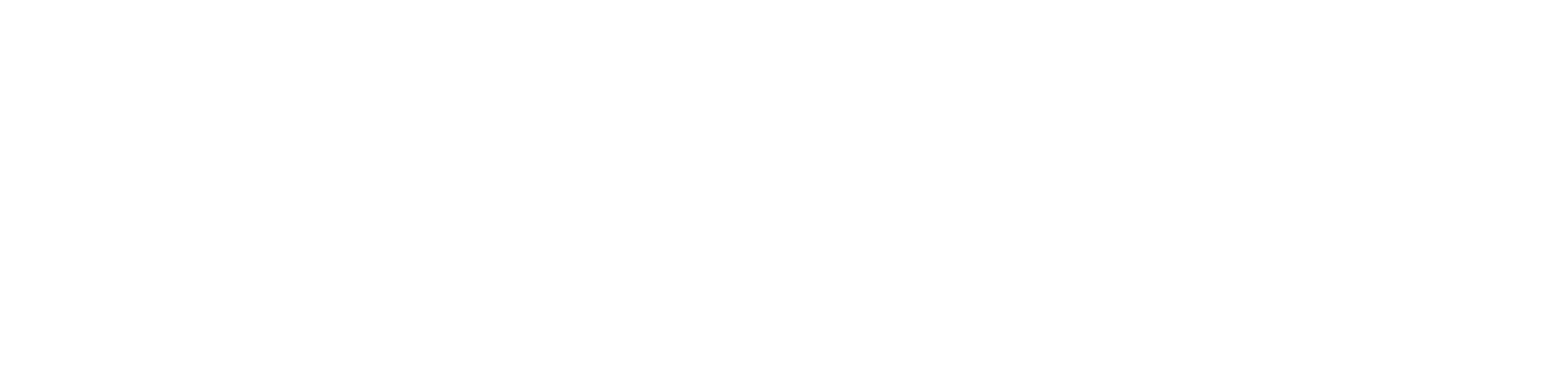
Evaluate and improve quality, enhance process performance.
- Monitor your processes using Shewhart charts in a whole new way with an interactive Control Chart Builder that lets you drag and drop to explore potential root causes of variation.
- Receive JMP® Live notifications for out-of-control signals from control chart warnings.
- Study the capability and performance of many variables at the same time to easily identify processes that aren't meeting expectations; drill into problem processes to identify potential root causes.
Statistical Process Control (Shewhart Control Chart)
- IR
- Xbar R
- Xbar S
- P
- NP
- C
- U
- CUSUM
- EWMA
- UWMS
- Multivariate control chart
- Western Electric rules
- Tests for special causes
Process Capability (Capability Index)
- Cp
- Cpk
- Pp
- Ppk
- Specification limits
- Goal plot
- Capability
- Performance
Quality Assessment (Process Screening)
- Stability index
- Quality review
- Shift detection
- Process performance
Measurement System Analysis (Gage R&R)
- Evaluate Measurement Process (EMP)
- Variability chart
- Linearity, bias
- Kappa
- Agreement
- Attribute Gage R&R
- Variance components
- MSA design
Quality Improvement (Six Sigma)
- Pareto Chart
- Cause-and-effect diagram (fishbone, Ishikawa)
Acceptance Sampling
- OC Curves
The integrated analysis that JMP facilitates is very helpful to the way engineers think – and think critically at a high level.
Xiao Zhenglin
Organizational Excellence Training Manager, STMicroelectronics
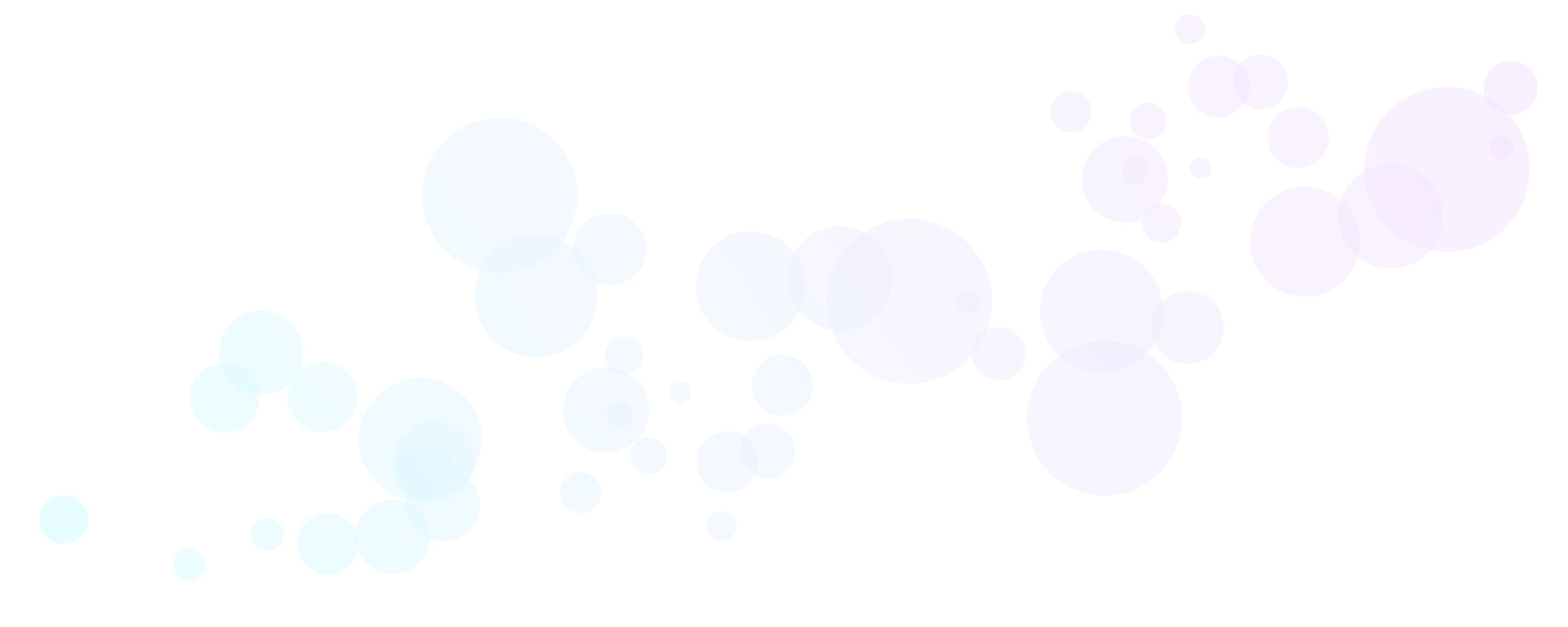
JMP® Analytic Capabilities
See everything that JMP® can do for you and your organization, from data access and cleaning, to exploration and visualization, all the way through sharing and communicating your results.
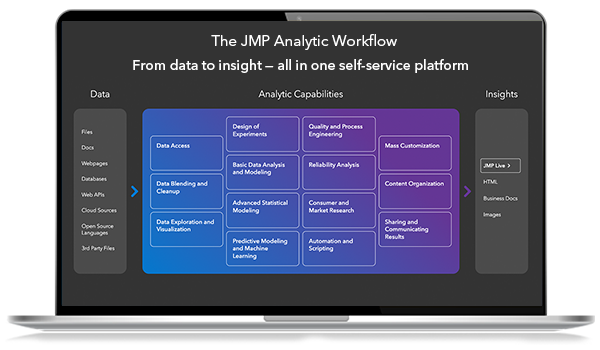