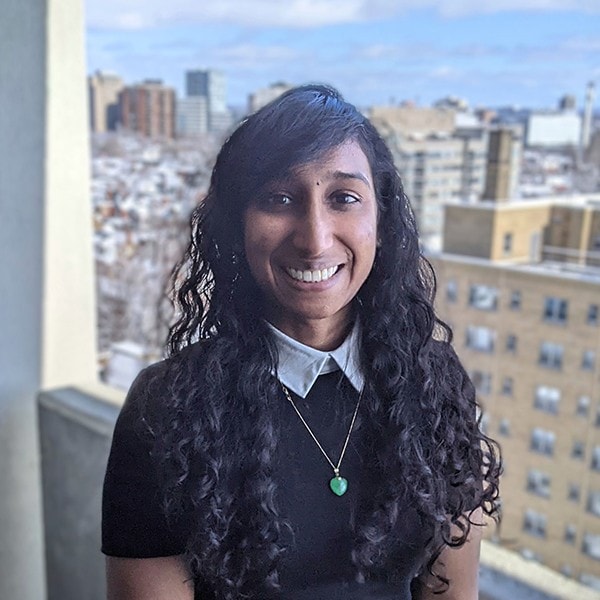
Levannia Lildhar
Data Scientist, Siemens Healthineers
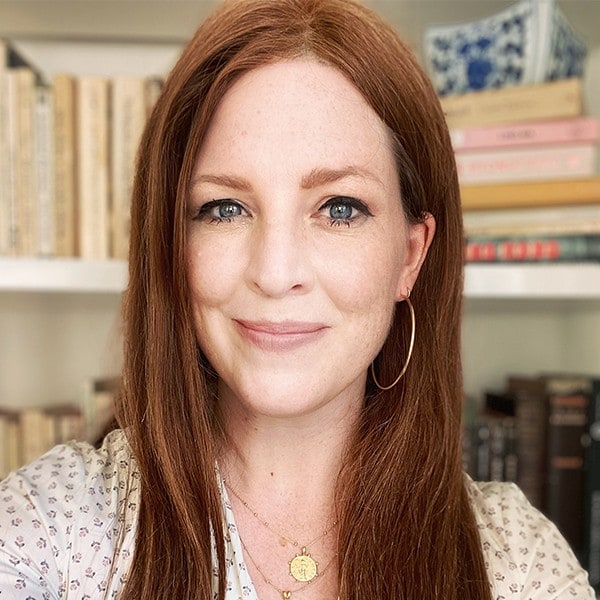
Meg Hermes
User Reference Manager, JMP
Levannia Lildhar is a data scientist for Siemens Healthineers based in Ottawa. A member of the company’s product engineering team, Levannia uses applied statistics to support work in field biosensor performance monitoring, calibration and validation of new R&D sensor technology.
She holds a master’s in data science from the University of British Columbia and undergraduate degrees from the University of Ottawa in chemical engineering and biochemistry. Her research has been published in the Journal of Genome Biology and Evolution and the Journal of Biomedical Materials Research. She is also a co-host of The Secret Life of Numbers, a podcast where she discusses numbers in everyday life.
Meg: Like so many of us today, your career path has been something of an evolution. How did you land on data science after starting off in chemical engineering and biochemistry?
Levannia: I began my career as a product engineer, which is a very classic role. We were building a product, helping increase yield, reduce scrap, conduct calibration – that sort of thing. But as time progressed, we started to realize that we were losing a lot of information by not analyzing our data to its fullest. We also started to wonder whether or not our models could be more robust and more future-proof.
At that time, though, I didn't feel like I had the skills to assess or build the models I needed. So I left Siemens Healthineers for 10 months to get my master's in data science. Now I’m back and working as a data scientist, and I’m really hoping to expand the purview of the data science team beyond its current scope: Can we build models that help the manufacturing line? Can we build models that look at automation or images, not just numbers?
With the knowledge I’ve gained, and together with the data science team, we've really been able to start laying down the foundations for broader data literacy. We’re moving toward “This is how we want to look at data, and this is the language that we use when we look at data.”
Meg: Do you think having worked in a product engineering role before moving into data science gives you a different perspective than, say, a traditional statistician?
Levannia: It certainly helps with communication. For example, when a data scientist makes a decision, they might base it on something that manufacturing or process engineering may not necessarily have been exposed to. They might be more concerned with the fact that the model works, why, and which key parameters they need to keep an eye on. That becomes your focus when you describe the work.
My engineering background also helps a bit when it comes to knowing what to work on or where to put emphasis. There’s a saying that all models are bad in some way, but some are useful. Having been a product engineer, I think it’s easier to see what will be useful. So I can make a bad model that is still useful!
Meg: Given the differences in how data scientists and engineers communicate, have you encountered much skepticism? What proofs of concept do you find are most compelling in the eyes of domain experts?
Levannia: I like to think of our data science team as serving all the other teams; our goal is to serve the entire organization. We're still pretty young because I think the idea of data science in healthcare is still pretty young. We're still learning where we fit in and advocating for it, and we still come across those individuals who don’t yet have much trust in some of the more advanced techniques that we may use. They still need to see it in action – which is fair because we build medical diagnostic devices that affect patients’ lives, so this type of careful approach is definitely warranted and needed!
I would say that on the whole, it can take time to accept more advanced techniques. We had to show that, if something went wrong, it wasn’t the more advanced technique that was causing the issue. It can feel like you’re always defending the analytics. But when you've gone through that process many times, you have the confidence when a problem arises that it is in fact a problem that should be solved, not just an anomaly in the model.
In many cases, we look at several situations and edge cases. And that requires spending time gathering the data and developing the proof that a new technique can perform both accurately and precisely. I say that laughing, but sometimes it can be frustrating when you’re asked to look at a certain situation and you're like, “Well, of course a model can do that.” It all comes back to communication.
As you’re becoming more advanced in your modeling techniques, you want to make sure that you’re communicating out what’s happening. And you have to realize that there are interesting situations that will be brought to you by domain experts who have been working within this context for a very long time. There may be something you hadn’t thought of. Doing this kind of work really tests you as well. But in the end, it also results in much more robust analytics and models, so I am also grateful for the scrutiny.
Lastly, it’s helpful to emphasize that a statistical approach or some of the more advanced analytics can save time, because people never have enough time! We can create models and build tools that will save time … and they’re often more robust. They can also help people get the information that they need to make decisions faster.
Meg: Has management been supportive?
Levannia: Yes. Though as with any team trying a new tool or approach, management wants to see what you can do first. We're still in the stage where [leadership at Siemens Healthineers] is giving us the freedom to see what we can do. And they point us in the right direction perhaps by giving us interesting problems to solve that will also help the business.
Right now everyone – no matter what industry you’re in – is learning that there's so much data everywhere, and that if you analyze it correctly, you can do a lot of things: Save money, become more predictable, become more reliable…. I think as organizations grow – and especially in healthcare with COVID – we've had to increase our production and think about how we can be more efficient. Even in areas like supply chain, teams are thinking about how data can be better used.
Meg: What role does training have to play as the field evolves?
Levannia: I don't think you need formal training to be a data scientist. People were doing data science long before we had this trendy term – it’s just that we've labeled it now.
That said, part of my role is to support training, and JMP is the tool that we use. Think about what people want to learn to do with their data at the start: They want to visualize it, label outliers, graph it, tabulate it. JMP does all of that very quickly and easily, and you don't need very much training to do it!
With JMP, you have the ability to move faster. Honestly, it's one of the best tools I've seen for data wrangling and data visualization. You don't need to download Python or R, and you don't need to understand how to code.
Meg: You mentioned the term “data literacy” and I’m wondering: What's your vision for Siemens Healthineers two to five years down the road?
Levannia: I hope I can inspire those whom I work with to think about their data more, and to think about their data literacy – I'd like it to be more of a common language: We use the same terms; we approach problems similarly; we understand what we need to consider.
Even with something as simple as data visualization, we can sometimes much more easily understand the difference between a problem and what is just noise. Also, we can integrate this way of thinking about the data into our processes so that it’s always there in the background. You can look at data from the beginning of a process to the end.
For example, if you can figure out which key indicators will cause failure, you can understand the trends before a failure occurs and proactively make adjustments. In the future, I would like our site – or our whole organization – to just think more about their data. Just think about it. Sit with it a while, maybe, play with it, build some graphs, and then start using it to help them answer questions. And we’re getting there.
Meg: Are you using any automation to help standardize best practices for approaching and thinking about data?
Levannia: Certainly, yes. At our site, there are two or three teams that use JMP heavily. We've created scripts that do repetitive work for us, and that's very useful. In JMP, you can just save a script and run it from somewhere else. And you can share it with other people – which certainly helps build capacity because, rather than doing something from scratch, you have an automated process ready to go. That's very useful.
Meg: I'm curious about your experience with the JMP Early Adopter program.
Levannia: I joined a couple of years ago, and I do like it because you can see the new features that are in development and comment on those features. Sometimes you see your comments come into play in new versions of JMP. And sometimes the things you want don't come out, and you're like, "Uh, I'm a little disappointed" – ha!
I think JMP does a very good job at keeping the conversation going. There is the [JMP systems engineering team] who are always there to help, and you can send them data. There are also seminars, and when we were having some issues with JMP 16, the customer service was very good. We would meet and show data, and you could interactively figure out what was going on and file bugs. I like that JMP has that back and forth with us because you feel that they’re actually working on your problems.
I was surprised as well that you can reach out so often and that the feedback is almost immediate in many cases. The [JMP online Community] forums were a great help to us at the point where we were starting to do more scripting. In fact, I should probably contribute to those forums more because they helped me so much and still do.
Meg: To wrap up, what advice you would give to someone who is just starting off – with their career, with JMP, maybe even with their education.
Levannia: JMP does a really good job with its training resources. There are some very, very good videos and training online – [Statistical Thinking for Industrial Problem Solving (STIPS)] is like a complete system right there for you. In fact, we ask our new hires to start by going through STIPS. If you're just starting out with JMP, that's a really good base.
If you want to be a data scientist, my advice is that you don’t need formal training. Curiosity about your data is all you need. In fact, a lot of people do data analytics work without realizing it. Anyone who cares about making data-driven decisions can call themself a data scientist. All you really need to do is spend more time with your data. And yes, you need to understand the tools and techniques too, and that really starts with tools like JMP that have made data science easy. Especially if you don't have formal training.
What it boils down to is: Don't be afraid to do interesting or bold things with data as you analyze it because you don't know what stories it can tell you if you don't look for them.