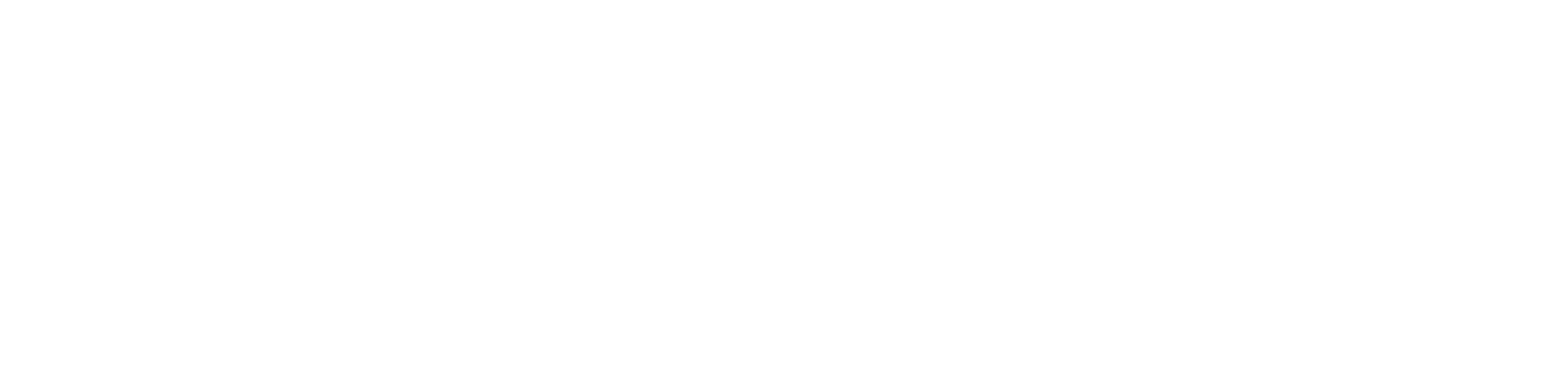
Advanced techniques that don't require an advanced degree.
- Easily deal with the diversity of modeling tasks: univariate, multivariate and multifactor.
- Use your data in the modern forms collected – text, functional and more – and transform it to data for building more useful models for better insights.
Multivariate analysis
- Principal components analysis (PCA)
- Clustering
- Correlation analysis
- Factor analysis
- Multidimensional scaling
- Structural equation modeling (SEM) PRO
- Discriminant analysis
- Multiple correspondence analysis
- Latent class analysis
- Partial least squares (PLS)
- t-SNE
Nonlinear modeling
- Dissolution
- Nonlinear regression
- Gaussian process model PRO
- Spline fitting
- Fit curve
Time series
- ARIMA
- Seasonality
- Autocorrelation
- Moving average
- Auto regressive (AR)
Advanced regression
- Generalized regression PRO
- Mixed models
- Generalized linear models
- GLMM PRO
- Penalized regression PRO
- Random effects
Variable selection
- Predictor screening
- Variable importance
- Stepwise
- All possible models
- Column contributions
Functional data analysis
- Spectral analysis PRO
- Wavelet analysis PRO
- Smoothing PRO
- Savitzky-Golay smoothing PRO
- Functional DOE PRO
- B-Spline PRO
- P-Spline PRO
- Fourier basis PRO
Process optimization
- Profiling
- Simulation
- Trade space analysis
JMP is the market leader. It utilizes strong multivariate tools, which we need, and every version release comes with new and improved bells and whistles.
Kieran O'Mahony
Data Science and Analytics Manager, Dairygold
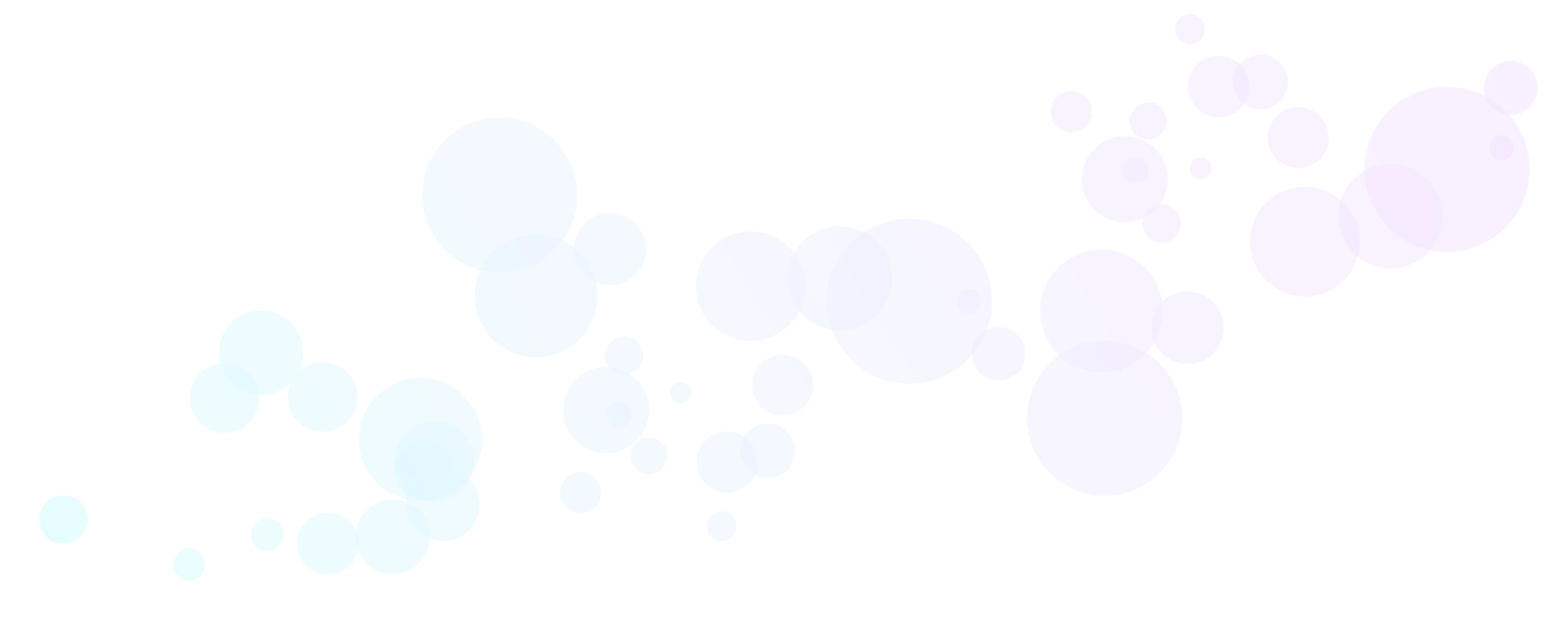
JMP® Analytic Capabilities
See everything that JMP® can do for you and your organization, from data access and cleaning, to exploration and visualization, all the way through sharing and communicating your results.
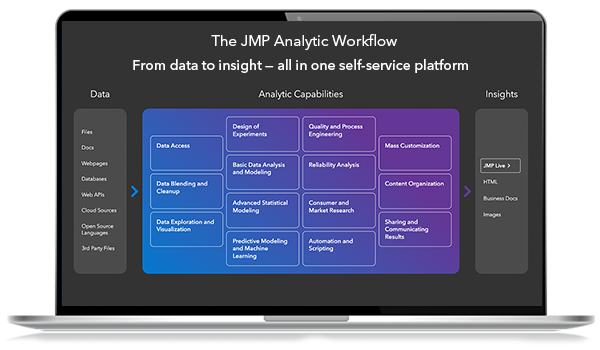