Jonathan Williams
Data Analysis Manager, IQE
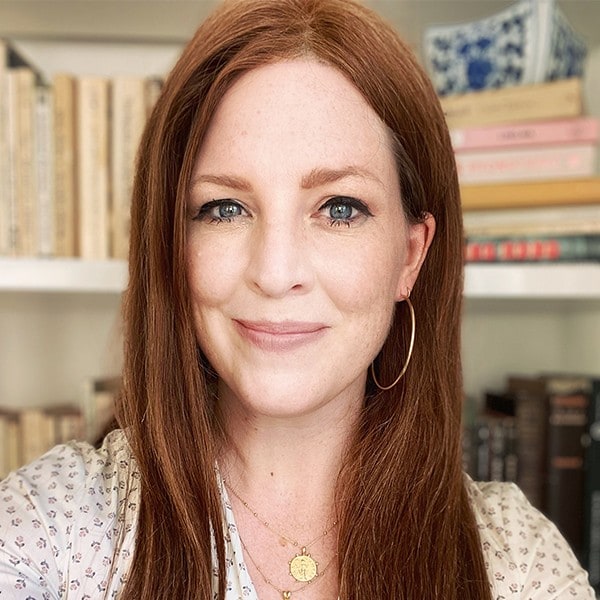
Meg Hermes
User Reference Manager, JMP
Jonathan Williams, PhD, is Data Analysis Manager at IQE, the leading global supplier of compound semiconductor epitaxial wafers based in Cardiff, Wales. Under his direction, the company’s Data Analysis team uses JMP® on a daily basis for data automation, operational process control, advanced statistical modelling and the development of bespoke analytical platforms.
Meg spoke with Jonathan about the many ways in which JMP has become an enabling tool in IQE’s analytics transformation.
Meg: As someone with an engineering background, how did you come to embrace industrial statistics?
Jonathan: I began my career in the semiconductor industry in 1996 as a process engineer in a silicon wafer foundry and later progressed to Module Manager. From the outset it was clear that the semiconductor industry used very large quantities of data from multiple sources, all of which are critical for producing a reliable, stable product.
An advanced statistical platform such as JMP was key to being able to manage and process a huge volume of information daily. As my experience in the statistical analysis of process data developed, I came to fully appreciate the power of JMP and its place in industrial statistics. I then moved into a data analysis role with IQE in 2012 to focus on this aspect of semiconductor manufacturing.
I see my role at IQE as transforming data into information; information that enables us to control our processing tools, increase our yield and performance and produce a quality product that meets our customers’ requirements.
Meg: Not every domain expert is keen to adopt statistical methods outright – in fact, we sometimes hear engineers voice concerns about statistical expertise supplanting professional intuition. What is your perspective on achieving the right balance between domain expertise and analytics in a company like IQE?
Jonathan: We are fortunate at IQE to have very experienced and knowledgeable engineers who have built up considerable process understanding over many years. However, personnel change over time and knowledge can be lost. By formalizing our data analysis, process control mechanisms and platforms through JMP, we ensure a continuity and consistency of approach throughout the organization with everyone using the same tools to manipulate the data in the same way. This provides stability to our toolsets and our product.
That being said, statistics cannot fully replace engineering insight into process interactions. For discovering and modelling interactions between the many process parameters, engineering knowledge of likely interactions are often essential in narrowing down the range of parameters, and an understanding of viable process domain space is needed to define sensible corners of a DOE.
There will always be a balance needed between professional intuition/insight and pure statistics, but JMP allows you to prove, quantify and refine interactions that otherwise would only be “believed” to be true.
Meg: Tell me about where IQE as an organization is right now when it comes to analytics enablement. How has the role of analytics evolved over the last few years?
Jonathan: The use of JMP across IQE has evolved tremendously over the last 10 years. From its core functionality of data mining and analysis by a handful of key users, JMP has become the standardized analytical tool for all users across multiple areas of the company. Its ability to import data from a wide variety of sources, either interactively or scripted, has empowered users of all abilities to quickly collate, process and report information that would have taken many hours to compile otherwise. The powerful graphing capabilities in JMP enable clear and compelling presentation of complex datasets, allowing data-based decisions to be made, leading to rapid problem resolution, faster cycles of learning and improved process control.
In recent years the Data Analysis team at IQE has developed a range of reporting platforms and data manipulation utilities using JMP add-ins and the JMP Scripting Language, JSL. This enables us to control analysis methodologies to ensure standardization and reproducibility across the various platforms and toolsets. The centralized library of scripted reports enables users to generate real-time comprehensive reports with configurable levels of detail, supporting high level oversite for senior management or detailed wafer or site-level analysis for the operational staff.
These improvements in our analytical processes are driven by IQE’s desire to provide world-class product to our customers with best-in-class performance, stability and detailed characterization. We work closely with our suppliers and customers to secure full end-to-end datasets, with detailed analytical and metrology data from our upstream suppliers, feeding into our internal process control and finished product characterization data and then feedback from device characterization data from our downstream customers.
In the semiconductor industry, we can have tens of thousands of data points per parameter per product item. This can result in very large relational databases to query and JMP has proven ideal for this purpose, allowing us to scale our operation without reducing our speed and efficiency. Once the power of analytical reporting via JMP became clear it was rapidly endorsed by IQE senior management and we have had positive engagement from users at all levels throughout the company.
Meg: I understand that prior to more widespread JMP implementation at IQE, most of your colleagues were using Excel, among other things. What were some of the primary shortcomings of existing systems that prompted the switch to JMP?
Jonathan: Prior to the rollout of JMP across IQE, data analysis was slow and time consuming.
Comprehensive data in our relational databases had to be extracted in small quantities through our MES, often manually copying and pasting small packets of data chunks at a time. This meant that datasets were inherently small-scale and subtle signals in the data, or the “bigger picture,” could easily be missed without significant time being spent first collating and then analyzing the available data.
Instead, JMP is able to quickly pull large quantities of targeted data from multiple datasets via multiple routes. Graphical and statistical analysis platforms provide rapid interpretation of the data and data mining to determine correlations is expedited by the Graph Builder and modelling platforms.
Excel has many virtues, but when querying large, complex databases, it does not provide the rapid flexibility of graphing that JMP does via various graphing platforms. Furthermore, Excel does not support design of experiments (DOE) or derive advanced statistical models without advanced programming skills. Once our users became familiar with JMP’s capabilities and ease of use, they rapidly migrated from Excel to JMP for most of their analytical needs.
Meg: What were the primary drivers behind the decision to adopt JMP more widely?
Jonathan: The speed, flexibility and power of JMP has enabled IQE to scale its operation without the additional resources that would otherwise have been needed. We are able to process, analyze and report more and bigger datasets, from more tools, for more customers, more quickly. Without JMP this would have required significant additional support.
The reporting platforms we have built in JMP have enabled users to run custom real-time reports on demand, supporting the operational requirements throughout the company. As such, we have staff at all levels making use of JMP’s capabilities.
Meg: What business impact is JMP having from a culture change perspective? Has it helped make analytics more accessible?
Jonathan: In short, JMP has enabled us to make data-driven decisions across the company. We are able to manipulate required datasets of the necessary size and content quickly and easily to provide clear graphical analysis. Reports are produced on-demand, presenting information clearly and concisely facilitating fact-based decisions. Issues are resolved quickly with clear direction from JMP, reducing downtime and improving customer quality. Full accessibility to our extensive databases and analysis in JMP has enabled us to provide a development route for future product, allowing us to model interactions and dependencies, directing future innovation and product development.
Meg: What business impact is JMP having from a technical perspective?
Jonathan: Speed and repeatability are two of the big benefits JMP brings to IQE:
Speed from the accessibility to our data, as and when we require it, tailored to the problem at hand; speed from the analysis and interpretation of the data; speed of reporting the data, thereby allowing rapid operational decisions.
Repeatability from our data manipulation utilities written in JMP; repeatability of analysis, providing consistency and stability of our processes and product.
Repeatability for automation of regular analysis and reports. We have large numbers of regular reports covering various timeframes that can be required daily, weekly, monthly or longer; some of these reports are internal but many are external. Analysis and reports must be produced on-time, regularly and consistently. JMP scripting allows for full automation of these processes, many of which could not otherwise be produced at the scale required in the time available.
Meg: What are some of the biggest challenges you face as an advocate for analytics enablement?
Jonathan: Historically, the biggest challenge for analytics enablement is that people would think too small. Before JMP, they had to work hard to obtain, analyze and present data in anything other than very limited datasets. As such they would request multiple iterations of piecemeal analysis gradually expanding and shifting emphasis as signals became apparent.
The power of data mining in JMP is ideally suited to working with bigger, statistically significant datasets. Over time we have succeeded in coaching users to more fully understand the analytical capabilities of JMP, so now they tend to ask for the world, but still look suitably pleased when they get it.
Meg: What were the most important lessons you learned in going through the process of advocating analytics transformation?
Jonathan: It is important to have senior management buy in when introducing a cultural change like JMP analytics. A JMP champion amongst the senior management will ensure JMP gets the high-level visibility that will ensure engagement throughout the workforce.
Secondly, JMP is so powerful that it can produce very technical analysis or very detailed graphical displays. Remember that sometimes much of your audience will not be statisticians. It may be worth producing a slightly simplified presentation for clear decision making, with backup slides for the highly technical information.
Try to encourage users to use the full power and scale of JMP. For users who have previously had limited access to datasets, there is a tendency to make small requests, often hiding data signals, making data interpretation difficult.
Automation of repetitive tasks is always well received. Try and identify repetitive frequent tasks that JMP can take care of for users. This will seed your analytics transformation at multiple points throughout the company, rather than relying just on the senior management team to champion change from the top down.
Meg: Is there anything you’re particularly excited about doing with JMP in the future?
Jonathan: We have recently acquired JMP Pro and advanced modeling capabilities are our main focus at this time. We have a number of statistical models derived in standard JMP and have been regenerating these in JMP Pro to verify, refine and validate the existing models. We have also been exploring the different modelling platforms within JMP Pro to identify further dependencies and correlations within our complex datasets. Several interactions have been modelled, and we are currently reviewing with a view to implementing them in our control processes.