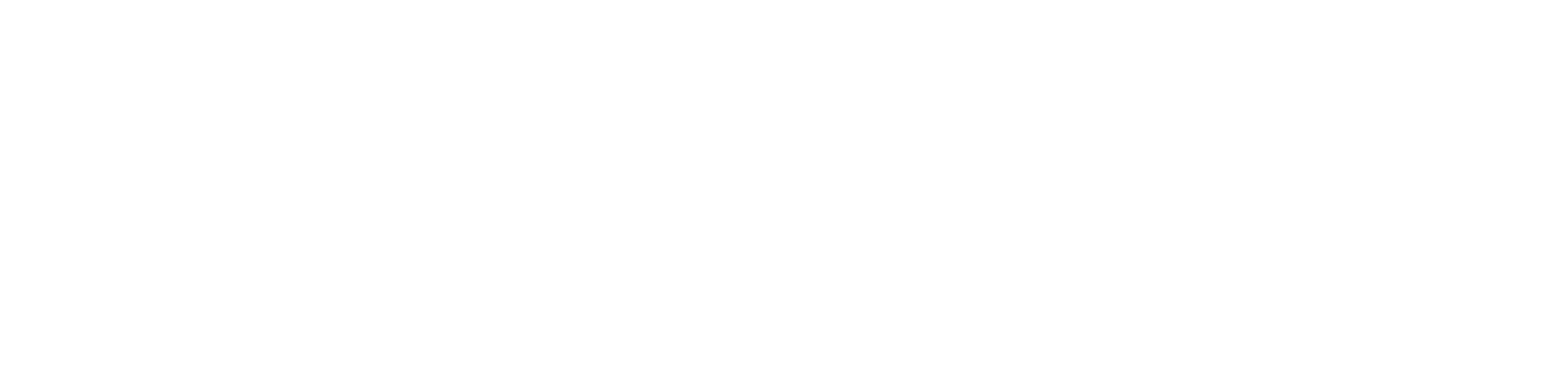
Consumer and Market Research
Better understand consumer insights to create better products and services.
- JMP provides a comprehensive set of tools for marketing and consumer research analysis. Use these findings to release better products, react to market trends, increase profitability, and build your competitive edge by understanding the voice of the customer.
- Bridge the gap between your customers, R&D teams, management, and sales through objective data rather than opinions or anecdotal evidence.
Statistical solutions have enabled us to extract more insight
with a smaller resource bank.
John Cunningham
Co-founder, PHYZZ
Segmentation
- Clustering
- Hierarchical clustering
- K-means clustering
- Self-organizing maps (SOM)
- Discriminant analysis
- Multidimensional scaling
- Multivariate embedding (t-SNE) PRO
Explanatory Modeling
- ANOVA
- Regression
Survey Analysis
- Latent class analysis
- Correspondence analysis
- Odds ratios
- Relative risk
- Item analysis
- Categorical data analysis
- Text analysis PRO
- Factor analysis
- Structural equation modeling (SEM) PRO
Text Mining (Text Analysis)
- Latent class analysis PRO
- Latent semantic analysis PRO
- Sentiment analysis PRO
- Term selection PRO
- Text regression PRO
Market Basket Analysis
- Association Rules PRO
- Affinity Analysis PRO
Experimentation
- A/B testing
- Choice PRO
- Conjoint PRO
- MaxDiff PRO
- Uplift PRO
Factor Analysis
- Structural equation modeling (SEM) PRO
- Exploratory factor analysis
- Confirmatory factor analysis PRO
- Path diagram PRO
Featured Resources
- Sentiment Analysis: What Are People Feeling?Mike Anderson discusses how sentiment analysis and term selection in JMP can help you gain insights from your survey text, social media and other forms of text data.
- Deriving Sentiments from Opinions or Product ChoicesSee how to combine text analysis with predictive modeling to derive sentiments from opinions or product choices to help understand the underlying emotions related to survey responses.
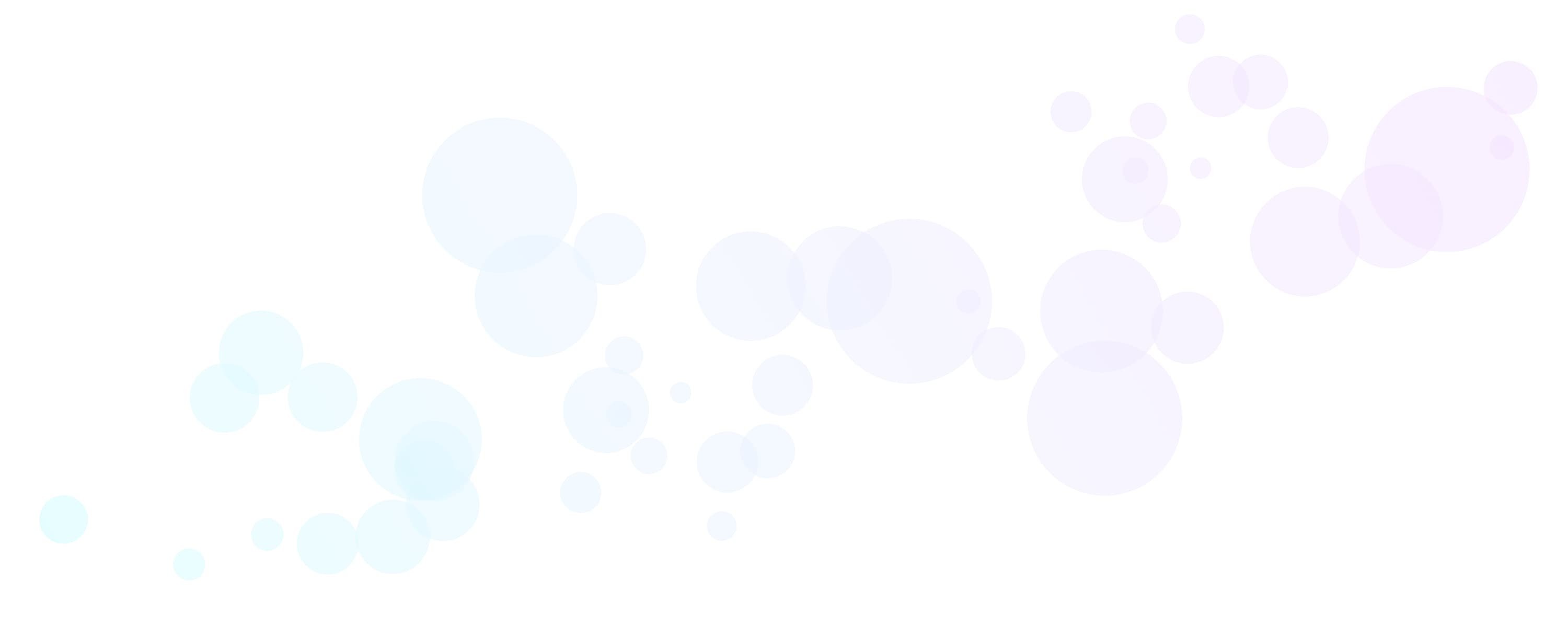
JMP® Analytic Capabilities
See everything that JMP® can do for you and your organization, from data access and cleaning, to exploration and visualization, all the way through sharing and communicating your results.
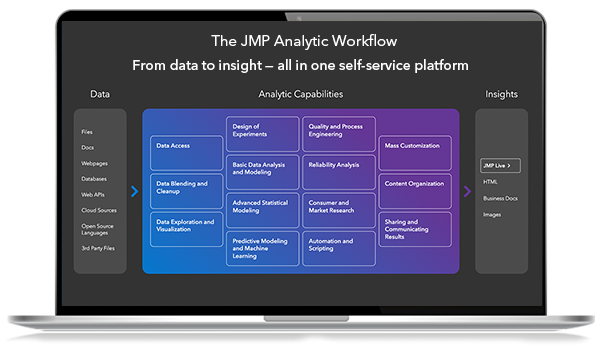